Generative AI is one of the most fascinating developments in artificial intelligence. It is a ground-breaking method that enables machines to produce new content—like images, text, and music—without the need for human input. The diverse applications of this technology are slowly becoming known to all, but how does generative AI work? Some of the applications and mechanics of this cutting-edge technology are covered below.
What is Generative AI?
Generative AI is a subdomain of artificial intelligence that focuses on creating models capable of generating new data samples that resemble the patterns present in the training data. Unlike traditional AI systems that are designed for specific tasks, Generative AI has the potential to produce content without explicit programming, harnessing the power of machine learning and neural networks.
The core idea behind Generative AI is to teach a model to learn the underlying distribution of the training data and then use that knowledge to generate new, never-before-seen examples that possess similar characteristics to the original data.
Generative AI vs Predictive AI
Generative AI and predictive AI are two distinct subfields of artificial intelligence that serve different purposes and employ different techniques.
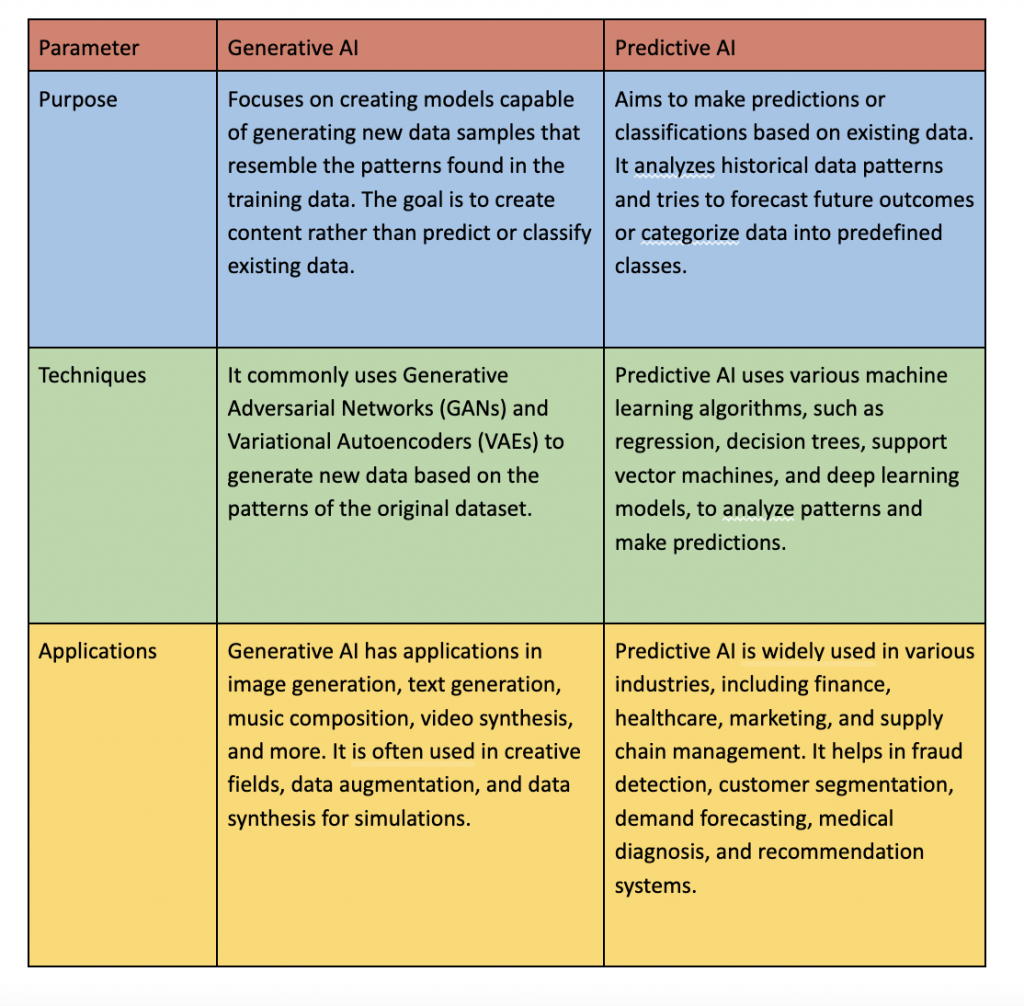
Applications of Generative AI
As many as 300 million jobs could be impacted by this most recent wave of AI technology, according to a Goldman Sachs report. However, generative AI has also uncovered intriguing opportunities in a number of different fields.
Source: Boston Consulting Group analysis
Here are some of the most notable applications:
- Image Generation:
One of the most visually stunning applications of Generative AI is the generation of realistic images. Generative Adversarial Networks (GANs) can create images of objects, scenes, faces, and even artwork. Artists and designers can use these AI-generated images as inspiration or as part of their creative process. Additionally, Generative AI has enabled data augmentation for training other machine learning models, leading to improved performance across various computer vision tasks.
- Text Generation:
Generative AI has brought about significant progress in natural language processing (NLP). Models like the GPT (Generative Pre-trained Transformer) series can generate coherent and contextually relevant text, imitating the style and tone of human writing. These models have applications in content generation, language translation, chatbots, and even creative writing assistance.
- Music Composition:
Musicians and composers have also benefited from Generative AI. AI-powered models can create new melodies and music pieces in different styles, allowing musicians to explore novel musical ideas. These models can also serve as musical collaborators, assisting artists in composing new pieces or generating background scores for various media.
- Video Generation:
Generative AI has recently advanced to the point where it can create realistic video sequences. This technology has applications in computer graphics, special effects for movies, and even video game development.
Challenges and Ethical Considerations
While Generative AI presents groundbreaking possibilities, it also comes with several challenges and ethical considerations.
- Bias in Data:
Generative AI models are highly dependent on the data they are trained on. If the training data contains biases or unfair representations, the generated content may perpetuate these biases, leading to unintended consequences.
- Misinformation and Fake Content:
Generative AI can potentially create realistic fake content, including images, text, and videos, which raises concerns about misinformation and deepfakes. This highlights the importance of responsible use and awareness of AI-generated content.
- Intellectual Property:
The question of intellectual property rights for AI-generated content remains unresolved in many jurisdictions. Determining ownership and copyright for AI-created works raises complex legal challenges.
Conclusion
Generative AI represents a remarkable leap forward in the field of artificial intelligence, unlocking the creativity of machines and enabling them to produce content that was previously the domain of human artists and creators. Through techniques like GANs and VAEs, machines can generate realistic images, texts, music, and videos, pushing the boundaries of what AI can achieve.
While Generative AI holds incredible potential for creative applications, it also demands careful consideration of ethical implications and responsible use. As the technology continues to evolve, we must strive to achieve a balance between innovation and ethics, ensuring that Generative AI enhances our lives while minimizing its potential negative impacts.
FAQs:
- What is Generative AI, and how does it work?
Generative AI is a subfield of artificial intelligence that focuses on developing models capable of generating new data samples that resemble the patterns found in the training data. Unlike traditional AI, which is designed for specific tasks, generative AI empowers machines to produce content without explicit programming. It employs techniques like Generative Adversarial Networks (GANs) and Variational Autoencoders (VAEs). GANs consist of a generator and discriminator, competing in a game-like scenario where the generator creates synthetic data and the discriminator distinguishes real from fake samples. VAEs use a latent space with a specific probability distribution, allowing controlled sampling and smooth interpolation to generate new data. Generative AI finds applications in image, text, and music generation, among others, but raises ethical concerns regarding bias, misinformation, and intellectual property rights.
- What is the technology behind Generative AI?
Generative AI works by using advanced machine learning techniques to train models on a dataset and learn its underlying patterns. The model is then capable of generating new data that resembles the patterns present in the original dataset. Two popular approaches for generative AI are Generative Adversarial Networks (GANs) and Variational Autoencoders (VAEs).
- Generative Adversarial Networks (GANs): GANs consist of two neural networks: a generator and a discriminator. The generator creates synthetic data from random noise, while the discriminator’s task is to distinguish between real data (from the training dataset) and fake data (generated by the generator). During training, the generator starts by producing low-quality fake samples that are easily discernible by the discriminator. As training progresses, the generator refines its output, making it more challenging for the discriminator to differentiate between real and fake data. The adversarial interplay between the two networks leads to the generator becoming proficient at creating realistic data that closely resembles the original training data.
- Variational Autoencoders (VAEs): VAEs are another generative AI technique. VAEs consist of an encoder and a decoder. The encoder compresses the input data into a lower-dimensional latent space, and the decoder reconstructs the data from this compressed representation. VAEs introduce a critical aspect: the latent space follows a specific probability distribution, usually a Gaussian distribution. This property allows the model to sample points from the latent space, which can then be passed through the decoder to generate new data samples. The controlled and smooth interpolation in the latent space enables meaningful exploration of the generative capabilities of the VAE.
3. What are some of the uses of Generative AI in banking?
Generative AI has found several valuable applications in the banking industry, including fraud detection and prevention through synthetic data generation for training robust models, generating personalized financial advice and investment strategies for customers based on their transaction history and risk profile, enhancing the customer experience by generating conversational chatbots for customer support, and automating document processing and report generation for improved operational efficiency. Additionally, generative AI can be utilized to predict market trends and simulate financial scenarios, aiding banks in making informed decisions and optimizing their services.